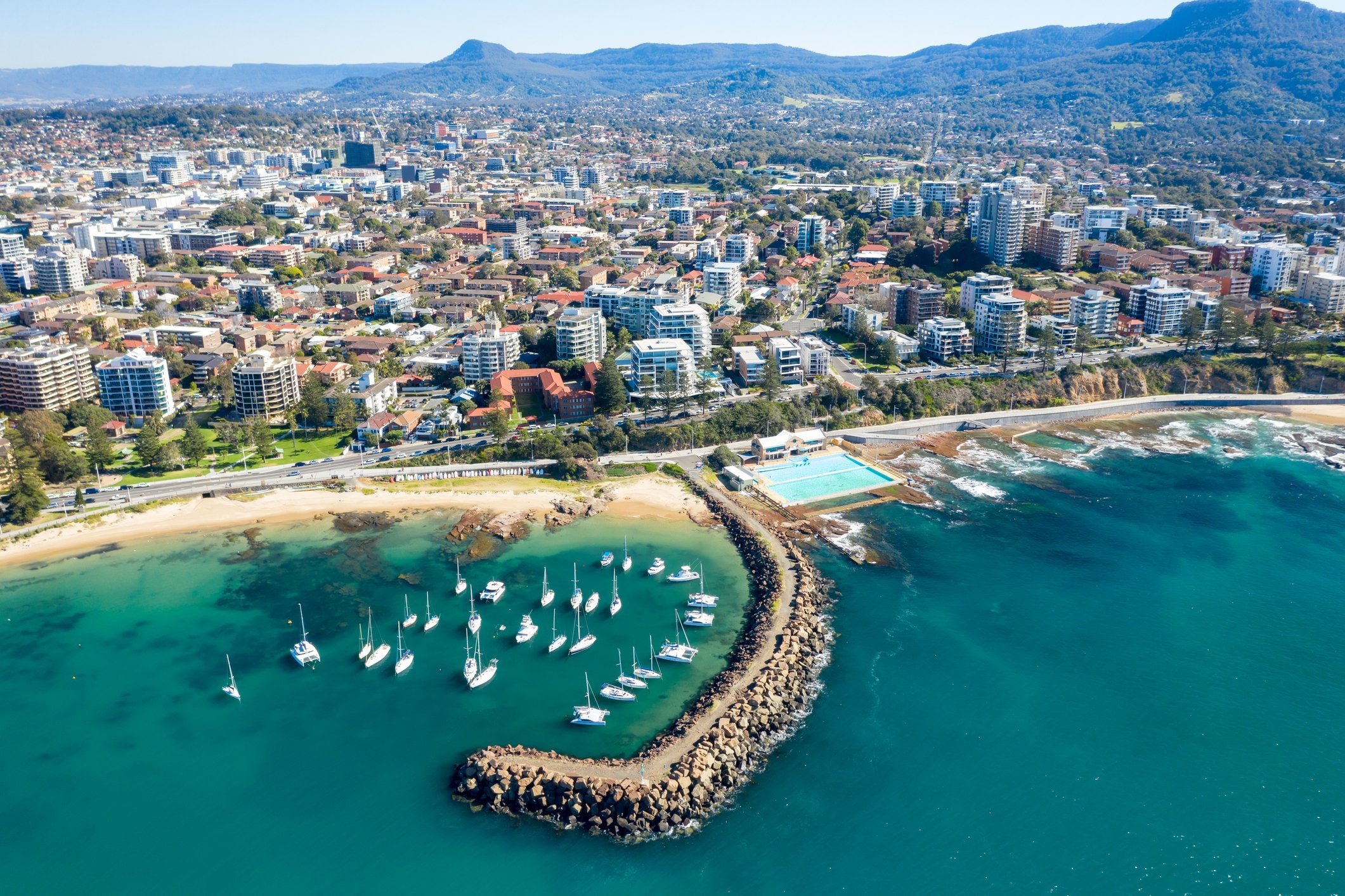
Meet the keynote speakers
ASC and OZCOTS 2023, 10-15 December 2023
Our Scientific Program Committee is preparing an incredible program for you. The following key-note speakers have been confirmed (with more to be added over the coming months - please check back from time to time):
-
Director, Poche SA+NT, Flinders University
Research & Education Lead Aboriginal and Torres Strait Islander Health, Victorian Comprehensive Cancer Centre Alliance
Kalinda is a Yawuru woman of Broome, born and living in Darwin. She is Director of Poche SA+NT at Flinders University and Research and Education Lead of Aboriginal and Torres Strait Islander Health at the Victorian Comprehensive Cancer Centre Alliance. Her research addresses the quality and governance of data that focuses on equity, the measurement of health disparities, and improving health services and outcomes. She holds a visiting fellowship at the Centre for Big Data Research in Health at UNSW and honorary fellowships at the University of Melbourne and Menzies School of Health Research. She is also Senior Editor at the First National Health and Wellbeing: Lowitja Journal and Deputy Editor of the Health Promotion Journal of Australia.
-
Department of Statistics, Harvard University, Cambridge, Massachusetts, USA
Xiao-Li is well known for his depth and breadth in research and his engaging and entertaining style as a speaker. His research interests include theoretical foundations of statistical inferences, statistical methods and computation, and statistical applications in natural, social, medical sciences and engineering. Xiao-Li was named the best statistician under the age of 40 by Committee of Presidents of Statistical Societies (COPSS) in 2001, and he is the recipient of numerous awards and honours for his more than 150 publications in at least a dozen theoretical and methodological areas, as well as in areas of pedagogy and professional development.
-
Professor Julie Simpson is Head of Biostatistics at the Melbourne School of Population and Global Health and Director of the Methods and Implementation Support for Clinical and Health research (MISCH) Hub at the University of Melbourne. She has 30 years’ experience collaborating on multidisciplinary research projects with clinicians, laboratory scientists, epidemiologists and health policy-makers at universities and hospitals (and even refugee camps) worldwide, and has published over 300 papers. Her main area of research is the integration of biostatistics and mathematical modelling to improve the control of infectious diseases and statistical methods for handling missing data. She is a lead investigator of ViCBiostat (Victorian Centre of Biostatistics), ACREME (Australian Centre for Research Excellence in Malaria Elimination) and IDDO (Infectious Diseases Data Observatory).
Title: “Bridging the gap: integrating statistical modelling and mathematical biology “
Abstract: The success of malaria control relies on the availability of highly effective antimalarial drugs that can significantly improve individual treatment outcomes. The widespread emergence of drug-resistant parasites now threatens the efficacy of first-line treatments, necessitating the urgent development of novel regimens and combinations of existing and new therapeutic agents to ensure adequate cure of malaria.
Addressing this challenge, biostatisticians and mathematical biologists often approach the determination of optimal treatment regimens from a different starting point. Biostatisticians primarily analyse clinical data to estimate the effects of different treatment regimens on patient outcomes. While this approach provides valuable insights into the investigated dosing regimens, it doesn’t provide an appropriate model for predicting patient outcomes under different mechanisms of drug resistance or explore alternative dosing schemes — a necessity for improving the control of infectious diseases. Mathematical biologists begin by developing a model for prediction that captures the biological mechanisms of the infection, such as the life cycle of the malaria parasite within the red blood cell. However, when these “mechanistic” mathematical models are expanded to incorporate treatment actions and patient immunity, they often become highly complex, impeding their validation against clinical data within a proper statistical framework.
This presentation will outline an interdisciplinary approach that brings together mathematical biology and Bayesian statistical methods, demonstrating how it can be used to determine optimal treatment regimens and how this work has informed WHO treatment guidelines for malaria.
-
Professor Sir David Spiegelhalter FRS OBE is Chair of the Winton Centre for Risk and Evidence Communication in the Centre for Mathematical Sciences at the University of Cambridge, which aims to improve the way that statistical evidence is used by health professionals, patients, lawyers and judges, media and policy-makers. He has been very busy over the Covid crisis. He presented the BBC4 documentaries “Tails you Win: the Science of Chance”, the award-winning “Climate Change by Numbers”. His bestselling book, The Art of Statistics, was published in March 2019, and Covid by Numbers came out in September 2021. His career highlights include appearing on Desert Island Discs in 2022, and in 2011 coming 7th in an episode of BBC1’s Winter Wipeout.
He was knighted in 2014 for services to medical statistics, was President of the Royal Statistical Society (2017-2018), and became a Non-Executive Director of the UK Statistics Authority in 2020. He is @d_spiegel on Twitter.
Title: "Trustworthy communication of data-derived evidence: what it is, and how we can get more of it"
Abstract: The recent pandemic has emphasised the key role played by evidence based on data. But how do we decide whether to trust all the claims that are made? Are the numbers being used to manipulate us? Using a wide range of examples, I will look at the way that statistics can be used to try and persuade audiences to think or act in a certain way, and contrast this with efforts to make communication 'trustworthy', by presenting balanced information that seeks to inform rather than persuade. Trustworthy communication should also acknowledge uncertainty and limitations in the quality of the underlying evidence (something sadly missing from ChatGPT).
But if authorities admit their uncertainties and present both the potential benefits and harms of innovations, won’t people distrust them? The opposite appears to be the case, and I will discuss results from randomised trials by my colleagues that suggest that current one-sided information actively decreases trust in those sceptical of the innovation who, ironically, are the very people whose trust is being sought.
I will finish by showing some examples of trying to do things properly, in particular relating to the benefits and harms of the AstraZeneca Covid vaccine, and list the questions that anyone should ask whenever you are presented with claims that are based on numbers.
Sponsored by Business Events Perth.
-
Associate Professor Margarita Moreno-Betancur established and leads an internationally recognised hub of innovation and expertise in statistical methods for observational studies, particularly in causal inference. Her integrated research program encompasses the development of innovative methods, collaboration in clinical and public health studies, statistical training for non-statisticians, and biostatistics capacity building.
After a BSc in Mathematics at Universidad de los Andes (Bogota) and an MSc in Statistics at Université Pierre et Marie Curie (Paris), she completed a PhD in Biostatistics in 2014 at Université Paris-Sud (Paris). She held postdoctoral roles in France (Inserm, Paris) and the Victorian Centre for Biostatistics (ViCBiostat, Melbourne), following which she has been competitively self-funded, first with an ARC Discovery Early Career Researcher Award (DECRA, 2019-22) and now an NHMRC Investigator Grant (EL2, 2022-26). In April 2022, she was appointed co-Director of the Clinical Epidemiology and Biostatistics Unit (CEBU) at the Murdoch Children’s Research Institute (MCRI) and The University of Melbourne (UoM).
She conducts methodological research in the areas of causal inference, missing data and survival analysis, to which she and her team have made significant and innovative contributions that are impacting the field of biostatistics and beyond, by influencing the design and analysis of health research studies. She and her team have collaborated on studies in a range of areas, particularly in the life course and social epidemiology, and provide important training and mentoring to non-statisticians. A highlight is an innovative and widely attended short course on methods for observational studies, with a particular focus on cutting-edge causal inference concepts and methods. She has made broad-ranging contributions to supervising, training and mentoring the next generation of Australian biostatisticians, at the Masters, PhD and postdoctoral levels. She is part of the steering groups of ViCBiostat, the MCRI's LifeCourse Initiative and the Master of Biostatistics at UoM.
Title: Causal machine learning in health data science
Abstract: The ultimate goal of medical and health research is to improve patient outcomes and population health. As a result, the overwhelming majority of clinical and public health research studies ask “causal” questions, concerning the effect of treatments, policies, behaviours and other exposures on health outcomes. In many cases, especially in the current era of data deluge, these studies rely on observational (non-experimental) data to address causal questions. Unfortunately, for a long time the statistics discipline largely shunned the possibility of causal inference beyond randomised trials, and instead focused on the development of tools such as regression models without clarity regarding their usefulness and limitations for addressing the causal questions that substantive areas continued to ask. In recent decades, however, the discipline has seen the rise of a new area focused on determining the settings and approaches that could allow causal inference from observational data. This talk will first provide an overview of some of the fundamental contributions of this statistical area to enable and improve the study of causality in health research, and then describe the role of machine learning within this causal inference paradigm, including recent methodological advances.
-
Marijke’s passion is connecting data and people. She is a Research Fellow at the Australian Red Cross Lifeblood. With over 10 years of experience in statistical consulting in academia, government, and industry, she prides herself on connecting with collaborators and use her skills to enhance the story behind their data. Throughout her career, she has been an advocate for good statistical practice and the importance of telling the story behind the numbers with confidence and impact. As an applied statistician, she is not afraid to get her hands “dirty” to gain more understanding of the data generation process.
Title: The tale of a career with large variance and a central tendency of promoting good statistical practice
Abstract: Starting out studying a degree in psychology, my career has taken many unexpected turns. Whilst it would be easy to state that this was always part of the plan, the truth is more like a random walk being the driver. I have counted moths and mice. I have been hooked up to machines. I have received electroshocks. And that all for the greater good of statistics.
In this lecture I will walk you through the varied path of my career, lessons I learned along the way and demonstrating that the career of an applied statistician can have large variance, but at the centre of it is the quest for improving statistical practice in all application areas.
-
Andrew Zammit-Mangion completed his PhD at the University of Sheffield, UK, in 2012, and is currently an Associate Professor at the School of Mathematics and Applied Statistics at the University of Wollongong, Australia. His key interests lie in spatial and spatio-temporal models and the inferential tools that enable their use in environmental applications. He has published extensively on several topics in this area, including dynamical spatio-temporal modelling; spatio-temporal point process modelling; Bayesian hierarchical modelling; multivariate spatial modelling; and approximate Bayesian inference. He has also released software packages that facilitate spatial and spatio-temporal modelling. Andrew has worked extensively in two subfields of the geosciences: (i) Antarctic processes and their contribution to sea-level rise, and (ii) trace gases and their transport in the atmosphere. His work in (ii) has contributed to a framework for estimating carbon dioxide sources and sinks from satellite data (coined WOMBAT – the WOllongong Methodology for Bayesian Assimilation of Trace-gases) that is the recipient of a 2023 prize by the Section of Statistics in Physical Engineering Sciences (SPES) of the American Statistical Association. Recently, Andrew has also been looking at novel ways to combine deep learning models with spatio-temporal models, and at ways to use AI to accelerate inference with these models.
Andrew was awarded the Cozzarelli Prize Class III (Best PNAS paper in Engineering and Applied Sciences) by the National Academy of Sciences of the US in 2013, a Discovery Early Career Research Award by the Australian Research Council in 2017, the Abdel El-Shaarawi Young Researcher's Award by The International Environmetrics Society in 2020, and the Early Investigator Award by the Section of Statistics and the Environment of the American Statistical Association in 2022. He is currently a member of NASA's Orbiting Carbon Observatory-2 (OCO-2) Science Team, and in 2019 he published a co-authored book with Distinguished Professors Christopher Wikle and Noel Cressie on spatio-temporal modelling with R.
Title: Fast statistical inference with neural networks and amortisation: Golden ticket or red herring?
Abstract: Neural networks can provide solutions to tasks that were inconceivable just a few years ago and have benefitted society in numerous ways. These benefits primarily stem from a property often referred to as "amortisation": Training a neural network usually requires significant effort and resources but, once trained, the network can solve similar problems repeatedly and rapidly with virtually no additional computational cost. Hence, the substantial initial training cost of training neural networks is "amortised" over time. Amortisation can also be used to enable fast inference with parametric statistical models: Once a network is trained using observational data as input and inferential statements (e.g., point parameter estimates) as output, the network can make inference with future data in a tiny fraction of the computing time needed by conventional likelihood or Monte Carlo methods. These amortised inferential tools have several compelling advantages over classical methods: They do not require knowledge of the likelihood function, are relatively easy to implement, and facilitate inference at a substantially reduced computational cost. In this lecture I will first give a brief review of recent work that has leveraged the property of "amortisation" in statistical inference. I will then evaluate the merits and drawbacks of amortised inference from a statistician's perspective and conclude by outlining the challenges that need to be overcome for these inferential tools to gain widespread acceptance.